Treescape Typology for Great Britain
A 'treescape' is a landscape containing woody features, which have ecological functions similar to woodlands. They include natural woodland, managed forests, trees outside of woodland and woody linear features. In Great Britain, we have good quality data on woods and forests, but we lack assessment of the whole treescape to understand its character and value. Through the Connected Treescapes project we created a typology to categorise the GB treescapes into 14 distinct types.
Our GB Treescape Typology represents an entirely new way of describing and understanding treescapes. It can be used to:
- Support natural capital assessment and ecosystem service valuation;
- Inform spatial decision-making;
- Contribute to new insights into the social and cultural values of our trees and woodlands.
View an interactive version of the GB Treescape Typology on the AgLand Viewer. (When in the AgLand Viewer, select “Treescape Typology GB” in the left hand map).
Scroll down this page to see Methods and Descriptions of the GB Treescape Typology.
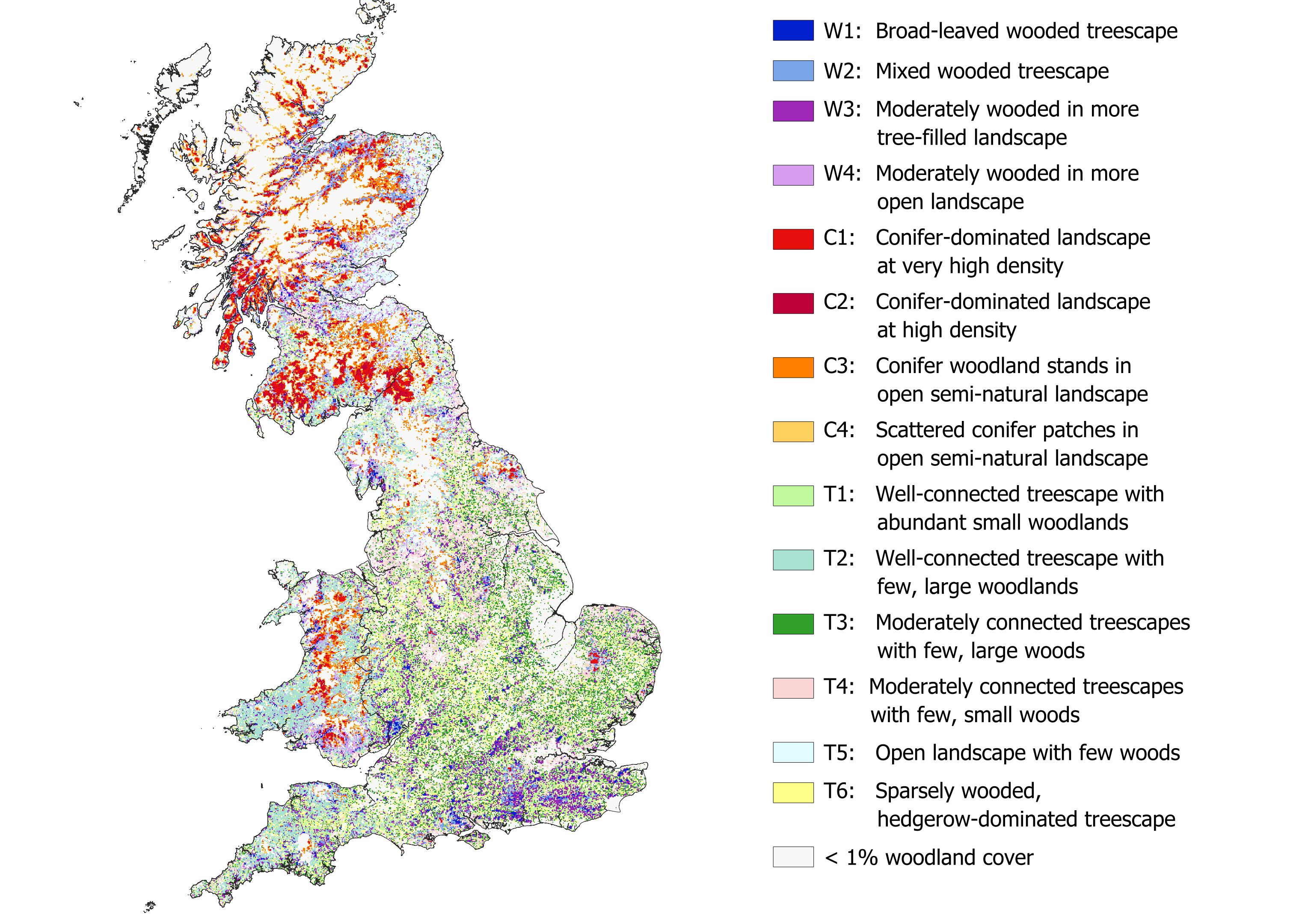
Method
We brought together 45 variables from 15 datasets treescape features, including quantity (e.g. woodland cover, tree cover density), quality (e.g. area of ancient woodland) and configuration (e.g. woodland patch size and connectivity) of treescapes with abiotic variables relating to climate, soil and topography. This included data on natural woodlands, managed forests, trees outside of woodland and woody linear features, which together form the whole ‘treescape’. We undertook clustering and dimensionality reduction using Self-Organising Maps (SOMs), which is an artificial neural network method to simplify and visualise complex data by reducing its dimensionality and grouping similar units into clusters, referred to as ‘nodes’ using the Kohonen R package. We ran 1000 iterations and selected clusters with >80% consistency. Analysis was carried out for all squares in Great Britain with <1% woodland cover.
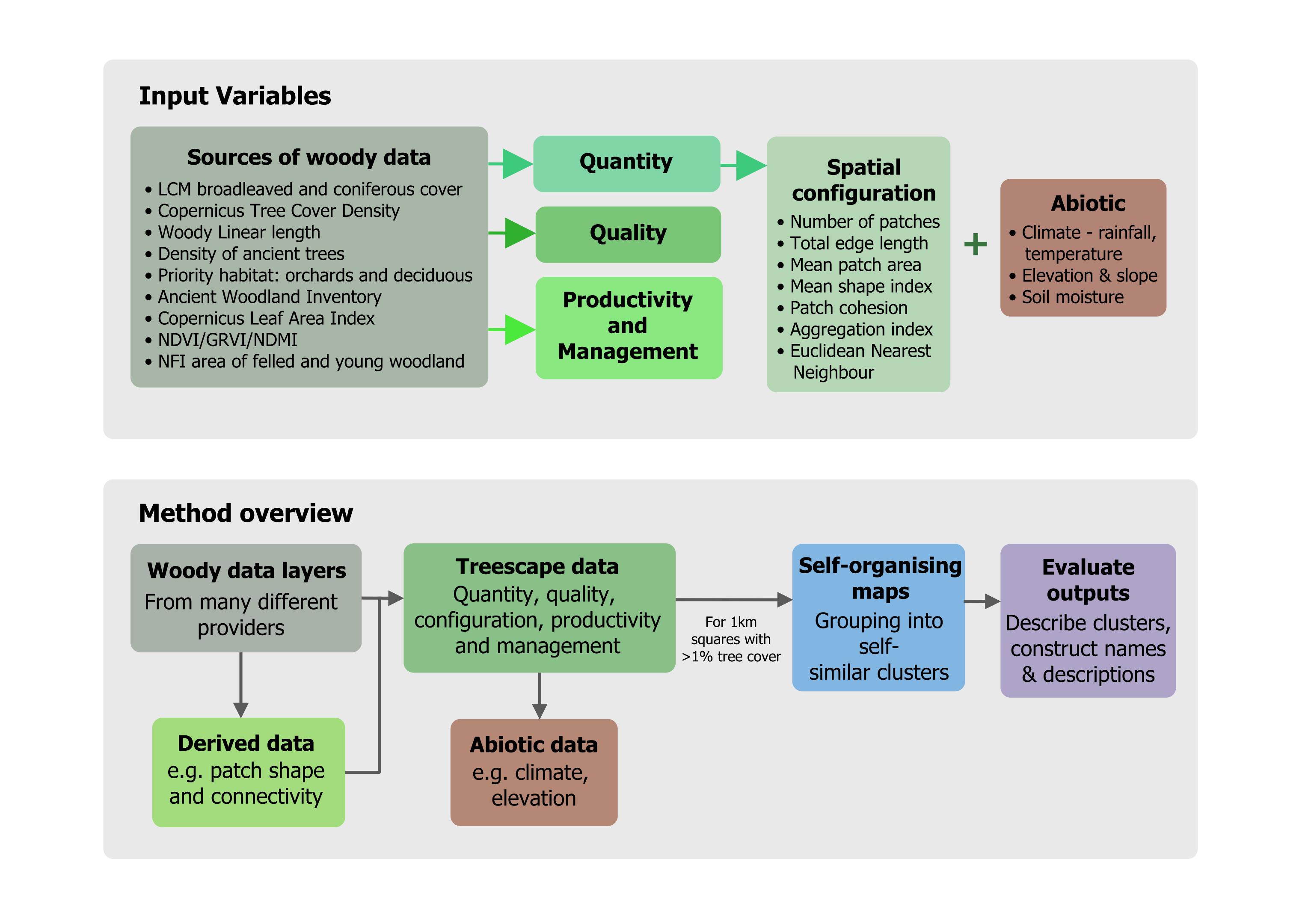
Creation of the treescape names
In order to come up with simple descriptions that adequately described the classes, and enabled related classes to be distinguished, we (i) examined the variable importance plots to identify key distinguishing variables for classes, (ii) examined the summary boxplots of the raw data to help describe distinguishing variables, and (iii) undertook exploratory cluster analysis to help describe critical differences between classes in a hierarchical way. In order for the model to run, we converted all the missing values (e.g. number of coniferous patches, when conifer cover was zero) to zero.
We used ‘rcart’ from the ‘rcart’ package in R to explore these clusters. We clustered the overall classes with all the variables directly focused on the treescapes, i.e. excluding broadly environmental variables such as elevation, NDVI etc. so that we could explore the distinct characteristics of these classes. In order to explore specific groups of similar classes in more detail, we ran the clustering with specific sets of classes alone to help discern the important differences between smaller groups. From this we created names and descriptions for each treescape. Below is a simplified version of the results of hierarchical clustering of the categories derived from the self-organised mapping analysis. It was produced to simply explain the relationships and differences between the treescape categories.
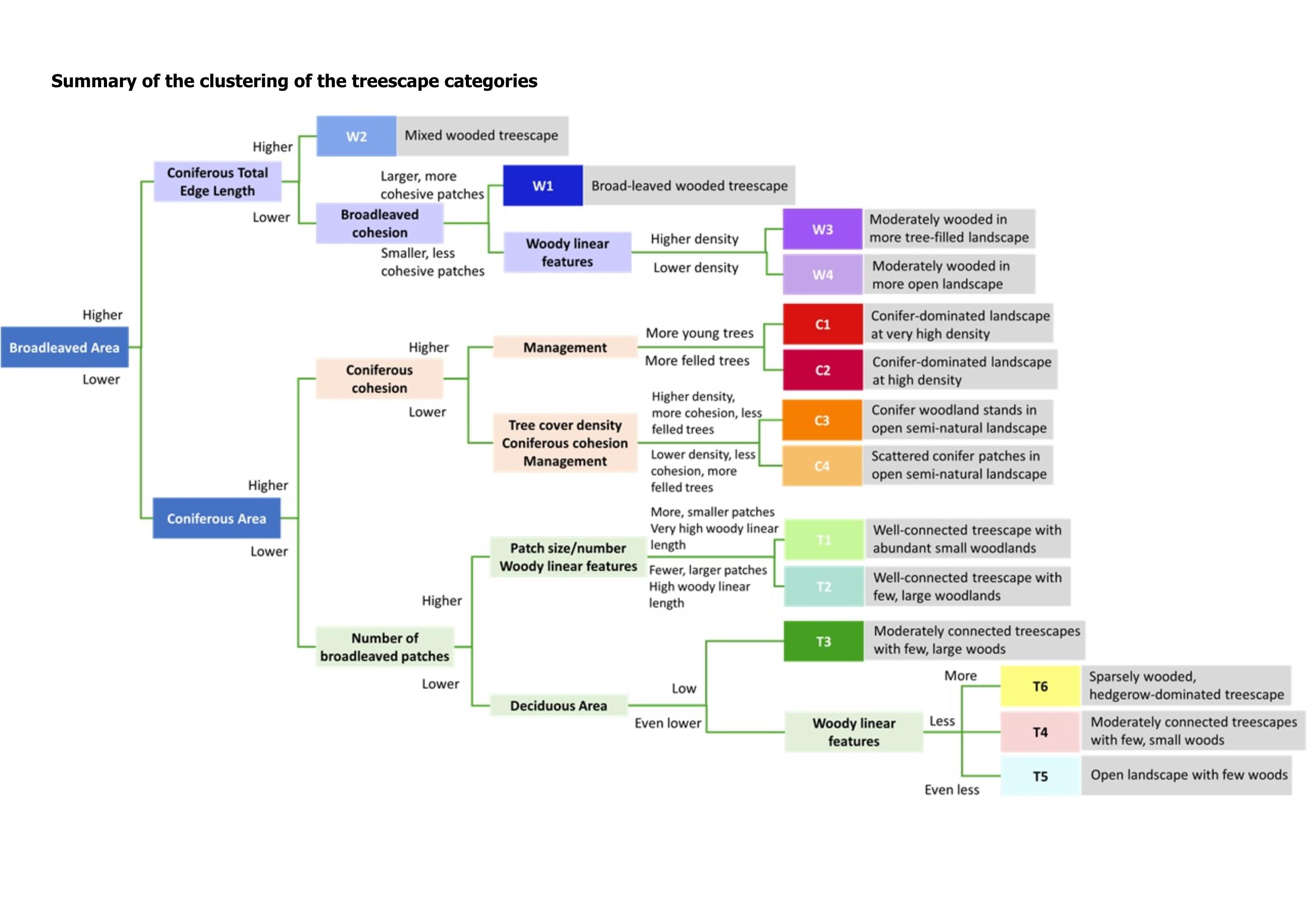